I want to introduce my theory on how we approach analytics. People often become captivated by numbers – while my idea is revolutionary, its practical applications are already well-documented in different industries.
Why data feels incomplete
Data has been at the core of decision-making for decades. Businesses, governments, and researchers rely on numbers to tell them what’s happening in the world, how people behave, and where to go next. But something has always been missing.
Numbers don’t tell stories. They don’t feel. They don’t experience.
Think about it — when was the last time a spreadsheet captured the feeling of frustration when a user abandoned an app? When did a customer retention report reflect the hesitation of a person deciding whether to stay with a brand? How often does an employee performance metric explain why someone is slowly disengaging?
The problem is simple: we treat data as if it exists in isolation, disconnected from human experience.
What if, instead of treating analytics as a passive observation of numbers, we treated it as a way to understand lived experience?
This is where experience-driven analytics comes in.
A phenomenological shift in analytics
In philosophy, phenomenology is the study of experience — not just actions, but how we perceive and feel those actions. It’s the difference between a fact and a lived moment. For more on the phenomenological approach, I recommend the article Husserl’s Phenomenology by
.Consider this example:
Fact: A user abandoned their cart after three minutes.
Experience: A user hesitated, re-read the price, had a moment of doubt, felt unsure, and left.
One is data. The other is life.
Traditional analytics sees the first. Experience-driven analytics strives to understand the second.
To do this, we need a new kind of system – one that doesn’t just track actions but captures meaning. You can read more about phenomenology in Data Science in the insightful article Rejecting Cognitivism .
Experience-driven analytics
Rather than treating all data as equally relevant, experience-driven analytics focuses on the moments that matter. This approach consists of three layers:
1. The broad view: seeing the patterns
At the foundation, we still need traditional data models — customer churn rates, engagement levels, product adoption curves. This is where we see the macro-trends that define general behavior.
But here’s the key: this level only gives us the outline, not the full picture.
2. The deep experience layer: identifying critical moments
Not every data point is equally important. Experience-driven analytics zooms into key inflection points – moments where emotions shift, decisions are made, and behavior changes. More about culture-driven analytics you can read in the article Model Positionality and Computational Reflexivity.
For example:
A sudden pause in user interaction before leaving a page.
A pattern of slower response times in workplace communication before burnout.
A gradual emotional tone shift in customer support messages before a complaint.
This is where machine learning meets phenomenology—by identifying moments that carry emotional weight.
3. The adaptive layer: learning and responding in real time
Instead of relying on fixed historical data, this model learns dynamically.
Using reinforcement learning, NLP-driven sentiment analysis, and event-driven architectures, the system doesn’t just describe experience – it adapts to it.
If a system recognizes hesitation in a checkout process, it can respond with tailored nudges. If an AI detects subtle disengagement in workplace interactions, it can suggest interventions before productivity drops.
This is the true power of experience-driven analytics – it bridges the gap between knowing and understanding.
Why this matters: from static data to living insights
When we move beyond raw statistics, we start to see patterns of human experience, not just events. A user doesn’t just “churn.” An employee doesn’t just “quit.” Behind every data point is a process of thought, doubt, engagement, frustration, and decision-making.
Analytics should reflect this depth.
Many industries are drowning in data but starving for insight. Companies chase more metrics, more dashboards, more reports—yet fail to understand the actual experience of their customers, employees, or users.
Experience-driven analytics offers a way to reconnect with the human element of decision-making.
Most AI systems today rely on prediction without understanding – they analyze trends but don’t perceive meaning. A new wave of AI must be designed to recognize experience, learn from it, and respond as humans would.
This is not just an advancement in analytics. It is a shift in how we see intelligence itself.
Examples: where this transforms industries
1. Customer Experience: Moving Beyond Surveys
Instead of waiting for post-interaction feedback, real-time experience tracking can adjust the user journey dynamically.
Emotional AI can detect and prevent frustration before it turns into churn.
2. HR & Workplace Analytics: Understanding Disengagement Before It’s Too Late
Employee engagement isn’t a score—it’s an evolving process.
Experience-driven HR analytics could detect early signs of burnout and disengagement before they lead to resignation.
3. Financial Decision-Making: Predicting Market Sentiment
Stock markets aren’t just numbers—they are a collective human experience of fear, greed, optimism, and hesitation.
AI-powered market sentiment analysis could transform how we predict financial trends.
4. Health & Well-Being: Personalized, Adaptive Support
Imagine mental health platforms that don’t just react to crisis moments but proactively recognize and adapt to shifts in well-being.
Experience as the new data layer
For too long, analytics has been trapped in the world of retrospective, linear thinking. But human experience is not linear. It unfolds, adapts, shifts. It is shaped by context, emotion, and meaning.
The question is no longer: “What happened?”
It is: “How was this experienced, and what does it mean?”
Experience-driven analytics doesn’t just provide better data. It provides a deeper understanding of how people think, feel, and act.
This is the next frontier of intelligence – where data is not just collected, but lived.
And in that shift, analytics will no longer be about tracking the past. It will be about anticipating the future, by understanding the present more deeply than ever before.
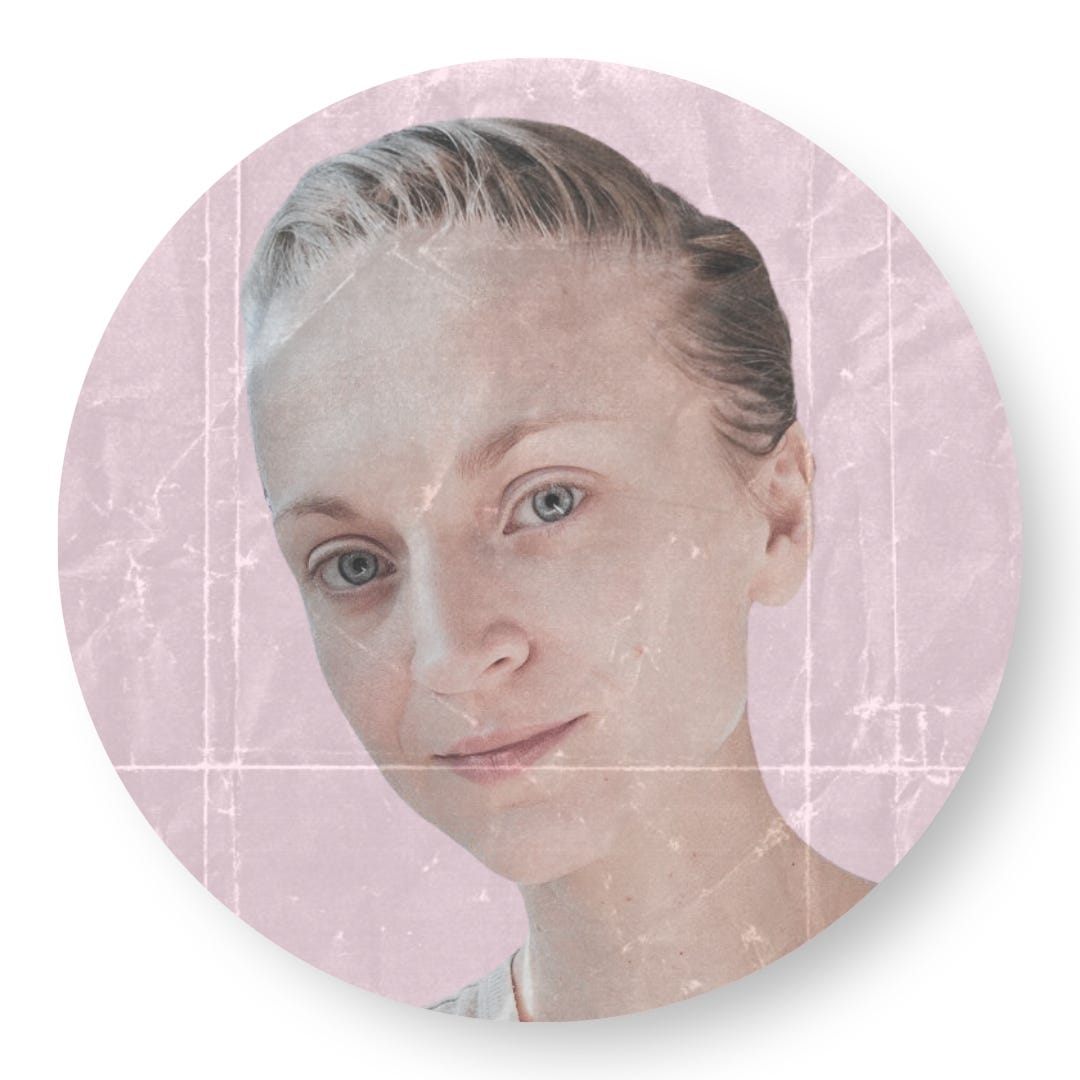
References:
Beckmann, P., Köstner, G. and Hipólito, I. (2023) Rejecting Cognitivism: Computational Phenomenology for Deep Learning. Available at: https://arxiv.org/abs/2302.09071.
Cambo, S.A. and Gergle, D. (2022) Model Positionality and Computational Reflexivity: Promoting Reflexivity in Data Science. Available at: https://arxiv.org/abs/2203.07031.
Husserl, E. (2020) Husserl’s Phenomenology: A Lo-Fi Voyage into the Essence of Thought.